Unlocking the AI black box
Design principles for transparent generative AI
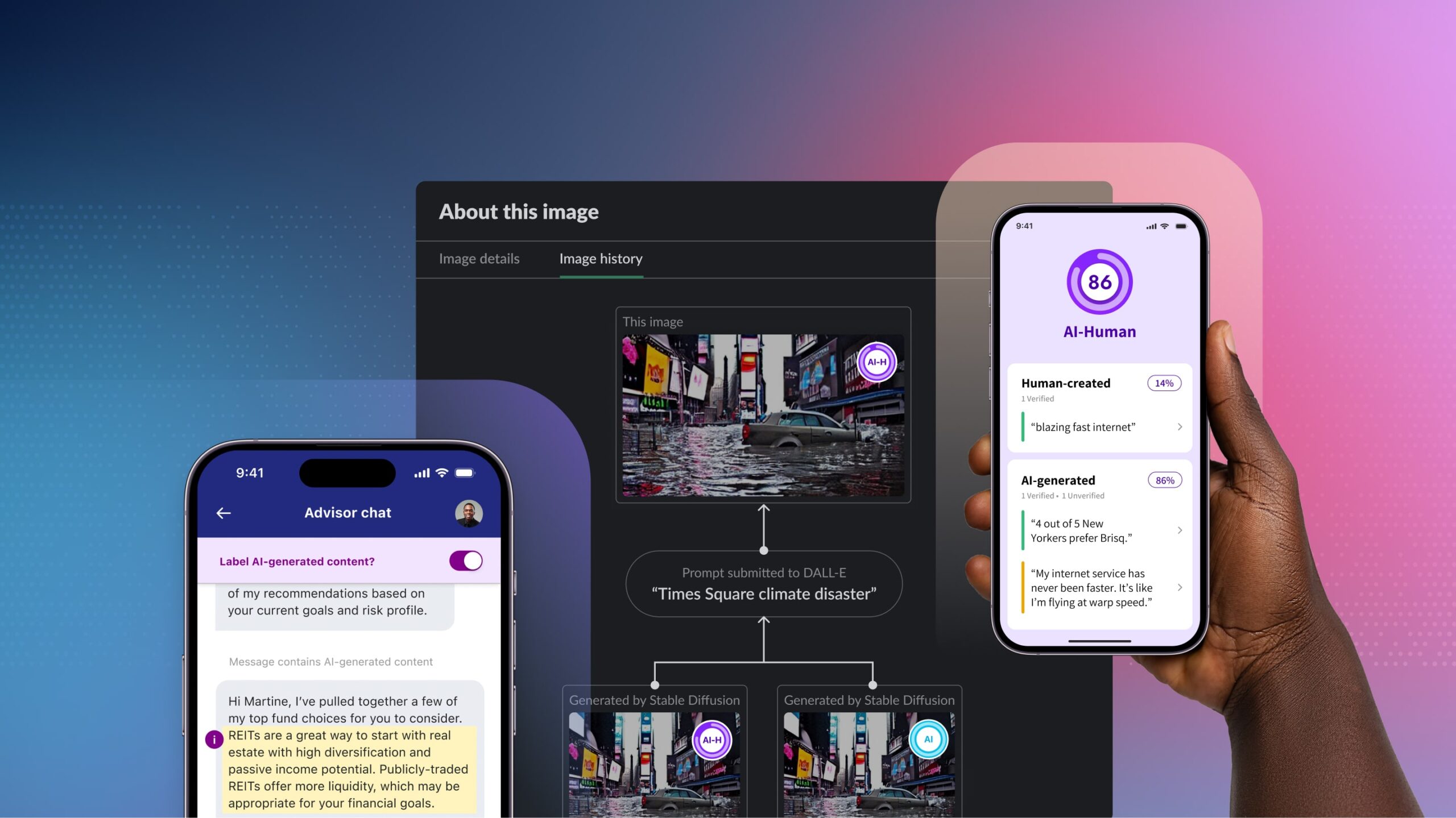
Envisioning
How can we make AI more transparent for users?
The Challenge
Generative AI, like many emerging technologies before it, has a trust problem rooted in users not understanding how it works and fearing the unknown. These tools can quickly spread misinformation, hallucinate, and amplify distracting informational noise that decreases digital wellbeing and reduces trust in systems that don’t authenticate outputs.
In partnership with the design editors at Fast Company, we explored a variety of contexts in which users may feel anxiety when encountering synthetic media content generated by AI. Our team explored potential UX solutions that could give users a greater sense of agency when interacting with synthetic content as it shows up in their lives.
The Outcome
We started by looking broadly at how AI-generated content might show up in our physical environments, such as in outdoor advertising claims, and how we might provide more transparency around such content at a glance.
We also considered how the integrity of synthetic content might be significant in a highly-consequential moment, such as when making a personal financial decision, when that is supported by a human advisor who uses an AI tool to provide recommendations.
Lastly, we considered how the line between what’s real and what’s synthetic can become blurred with generative AI, exploring how digital provenance and filtering tools might provide users with more agency and insight into controlling the flows of information in their field of view.
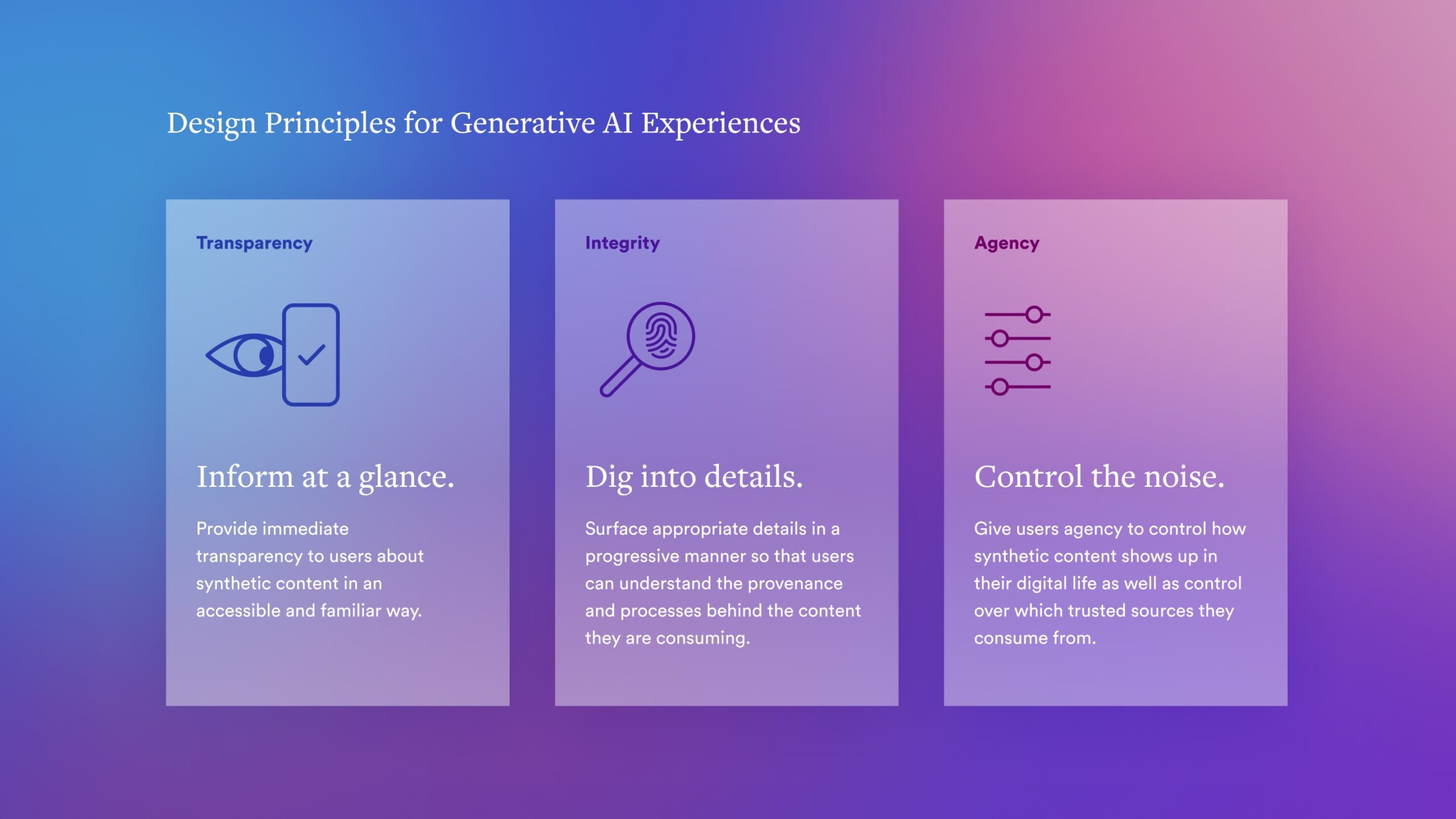
Creating a standardized label for AI-generated content
We explored how a simple, recognizable icon could help people to quickly discern between AI-generated, human-created, and AI-human hybrid content. Drawing inspiration from other rating systems such as film and TV ratings, we aligned on a simple three-grade system of codes: AI for 100% AI-generated content, AI-H for some balance of both AI-generated and human-created, and H for 100% human-created.
A simple blending of colors brings the ratings to life with two opposing hues: red for human—signifying our human vitality—and blue for AI— a commonly associated color with trust. When mixed together, the colors create a purple hue, which signifies the blend between human and AI-generated content.
The rating system is designed to provide recognizable and repeatable affordances for informing users at-a-glance. It’s usable across both digital and physical contexts, presenting as digital tag on devices or as QR codes in physical media. Details within the ratings provide users with progressive disclosure of the content’s origin and authenticity.
“[Users] want to maintain control over their experience. We were interested in the idea of giving users more affordances to have that sense of control, because that feeling of trust is not being garnered in the same way it is with a human.”
Authenticating media messages in our everyday environment
While synthetic media is showing up extensively on social media and in other digital spaces, we took our envisioning a step further and considered a future where AI-generated content is more prevalent throughout our daily information ecosystem, particularly in our physical environment.
Imagine arriving at a bus stop and seeing a digital display ad for a local internet service provider. While a glowing review of the service may seem inviting to a potential customer, the real story may be different. An AI system could present a dubious reframing of the training data of customer reviews, which may obfuscate the real story about the service.
By placing the standardized AI-H label on the advertisement, the ad’s audience has immediate context about the synthetic nature of the marketing copy. Scanning an associated QR code allows a user to instantly pull up information about the AI-compiled copy to see details about the sources.
A little more digging reveals that the internet service quality turns out to be quite poor despite how it is framed by the AI. Providing this type of transparency is critical to helping users feel more empowered in a landscape of increasing misinformation that could potentially be magnified by unethical or poorly-trained generative AI systems.
Providing clarity and assurance in consequential decision-making
Beyond marketing messages, generative AI tools can be used in back-end processes that support service delivery by humans. We were interested in exploring how AI might be used to support the recommendations of a human advisor in a highly-consequential use case like personal finance.
Imagine chatting with a human financial advisor as part of a robo-advisor service. As detailed, bespoke financial analysis and advice may not be appropriate for a client with a lower level of investments, the human advisor might leverage generative AI to source and compose question responses for the client before reviewing it and passing it along imbedded into that human advisor’s chat-based recommendations.
Since these back-end processes can be opaque to the end-user, clear labeling and disclosure built into the chat interface can help make the advice more transparent. In-context label and filtering affordances provide clarity at the moment of content consumption. Tooltips provide details about data sources, AI models used, and any relationships between recommendations and the model provider that could present conflicts of interest.
Provenance and filtering options for the personal information environment
As synthetic media proliferates, the digital informational noise that’s created has the potential to negatively impact users’ digital wellbeing. The inconspicuous blending of synthetic and human-generated content may become increasingly hard to discern, causing information overload, desensitization, and fatigue.
We explored how synthetic content might show up in a work environment, such as sharing an AI-generated news image on an internal messaging platform. While the imagery may cause initial alarm, such as seeing a natural disaster overtaking over a major city, providing the user with detailed metadata about the image, including a provenance tree and prompt history, reveals the true nature of the content—it’s synthetic.
However, source details may not be sufficient to control the digital informational noise of synthetic media. Users in highly-regulated or sensitive environments, may desire further control over the sources of synthetic content. We explored how filtering could be integrated at the OS-level, providing users with the highest-level of control over the information that appears in their field of view.
Ultimately, the user should be empowered to decide how algorithms shape their reality, yet often those algorithms are opaque and trained on questionable datasets. Ensuring that media sources can be authenticated and independently verified is a critical architecture for building trust between humans and AI-powered systems.
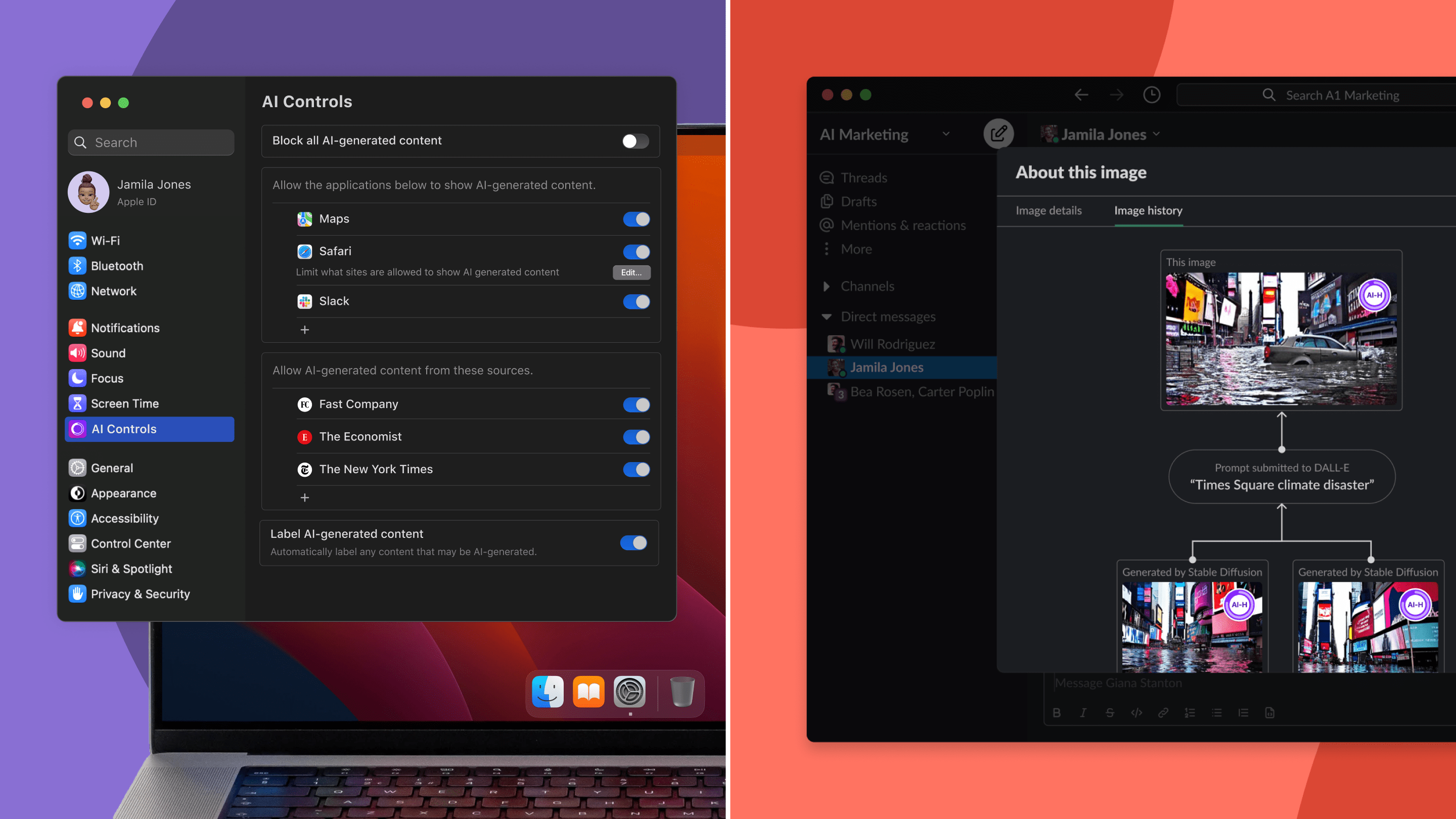
Read Next: